- The Daily AI Show Newsletter
- Posts
- The Daily AI Show: Issue #31
The Daily AI Show: Issue #31
Do you have synthetic memories about AI?

Welcome to the first of many 2025 editions of the Daily AI Show Newsletter.
In this issue:
Our 2025 AI Predictions
20 Can’t Miss Prompting Rules for 2025
Unlock Your Potential: Smarter Goal Planning with O1 and Gemini
Plus, we discuss Microsoft’s costly AI plans in 2025, AI synthetic memories—healing recollections or thought manipulation?, how Florida is doing Florida things with AI, and all the news we found interesting this week.
It’s Sunday morning
The holiday break is over, but hey, AI never took one.
Try not to let that stress you out.
The DAS Crew - Andy, Beth, Brian, Eran, Jyunmi, and Karl
Why It Matters
Our Deeper Look Into This Week’s Topics
Unlock Your Potential: Smarter, ‘Thinking’ Goal Planning and Pursuit with O1 and Gemini
AI reasoning models like OpenAI’s O1 and Google’s Gemini 2.0 Flash have deliberative planning capabilities, and are changing how individuals and businesses approach complex problem-solving. These "thinking models" go beyond standard chat interactions by processing your prompts with deeper reasoning, breaking down high-level goals into step-by-step strategic plans to achieve the objectives. While they may take longer and cost more to run than standard models, their ability to think through multiple layers of a problem, evaluate different approaches, and resolve on an optimum path makes them ideal for tackling complex tasks.
These models work by dynamically adjusting how much "thinking power" they apply to a task. Simple questions might return near-instant responses, while more complex prompts—like creating a multi-step business strategy or working through scientific reasoning—can take significantly longer as the model iteratively refines its output. This ability to generate multiple plans-of-action, evaluate results and iterate to a solution makes them powerful tools for setting and achieving personal or professional goals. Just confide to your Thoughtful Assistant what you dream of doing or becoming!
WHY IT MATTERS
Advanced Planning Capabilities: O1 and Gemini can break down complex goals into smaller, actionable steps, making them ideal for project planning, personal development, and business strategies.
Deeper Reasoning Power: These models handle complex decision-making better than standard language models by processing information iteratively and providing more comprehensive solutions.
Use Case Flexibility: From diagnosing multi-symptom health issues to planning a product launch, thinking models excel in tasks requiring layered analysis and contextual understanding.
Clear Goal Definition Required: Success depends on clearly defining goals and resources upfront. Models perform best when provided with a concrete objective and relevant context.
Accessible Yet Specialized: While these tools offer impressive reasoning capabilities, their slower response time and higher costs make them best suited for tasks where deeper analysis is necessary.
Our 2025 Predictions
The DAS crew made our predictions for 2025 this week. Here is what we came up with along with some audience predictions from our live YouTube chat.
Andy
Agentic AI Collaboration: The emergence of "crews of agents" where multiple specialized AI agents work together on tasks instead of a single multi-purpose agentic assistant. Replit Agent is already a council of expert agents who together fulfill on full-stack design and engineering tasks.
Mixture of Agents Services: Major companies will deliver "mixture of agents" services, marketplaces with selectable specialized agents to collaborate on tasks for end-users. And some companies will hire out their award-winning specialist agents via API to cooperate on the intelligence network.
Autonomous AI Development: Autonomous AI will be allowed self-improvement, contributing to its own development, moving closer to AGI, though not fully comprehensive yet.
Legal Industry Disruption: AI will increasingly replace tasks traditionally performed by lawyers, such as contract review and document analysis, leading to major shifts in the legal profession.
Brian
Agentic Workflows Expansion: More agentic workflows will emerge but will remain limited to structured tasks rather than full autonomous decision-making.
OpenAI's Walled Garden: OpenAI will continue expanding a "walled garden" strategy with agentic workflows to keep users within its ecosystem.
Hyper-Personalized Education Platforms: 2025 will see a significant rise in hyper-personalized education platforms using AI, especially for long-term academic support and curriculum tracking.
AI in Wellness Devices: AI-driven physical wellness devices, such as robotic massage systems, will expand in availability and capability, particularly for health-related technologies.
Jyunmi
Edge AI Growth: Significant expansion in edge AI applications due to new hardware advancements, especially in consumer electronics like smart home devices (e.g., Ring doorbells, smart fridges).
Major AI-Related Incident: A significant negative AI-related event (e.g., data breach or misuse by bad actors) could spark public backlash and stricter regulations.
Beth
Light-Based Chip Production: U.S. or other non-China manufacturers will begin producing light-based chips in 2025, though the cost will initially be high.
Neural Infrastructure Chips: Specialized chips designed for AI language models will gain traction over the currently gaming-focused Nvidia GPUs.
AI Polarization: Growing divide between strong supporters and vocal critics of AI, with oversimplified narratives dominating public discourse.
Blockchain in Notarization: Blockchain and AI will challenge traditional notary roles and document verification, potentially reducing the need for human witnesses.
Karl
Voice AI Expansion: Voice-based AI interfaces will see a 25-30% increase in usage due to their convenience in both personal and business contexts.
Enterprise Roles for AI Leaders: More internal AI-related job roles will emerge in enterprises, moving beyond technical roles into operational and strategic positions.
AI in Healthcare Breakthroughs: AI models like GPT-4 and beyond will likely contribute to significant medical breakthroughs, including the cure of a major disease.
Audience Predictions
The Daily AI Show's Growth: The show will become a go-to source for millions of viewers.
Anthropic's Leap: Anthropic will release an AI model that rivals or even surpasses OpenAI’s GPT-4o and o1.
Personalized Medicine: AI will drive personalized treatments and breakthroughs, especially in rare diseases.
Small Business AI Adoption: Increased use of AI tools by smaller businesses for specialized use cases, rather than mass adoption across all sectors.
Blockchain for Privacy: AI and blockchain will merge to help individuals maintain privacy from mass surveillance.
20 Prompting Rules for 2025
Clarity and Specificity Are Still Key:
Provide clear, concise instructions.
Avoid vague terms and use precise language.
Short declarative sentences work better than long, complex ones.
Break down prompts into smaller, separate tasks for better results.
Test Simple Prompts First:
Start with a basic prompt and adjust iteratively.
If results aren’t satisfactory, refine the prompt further rather than over-engineering it upfront.
Use Examples Over Descriptions:
Providing examples often works better than trying to describe the desired output with words alone.
Example-based prompting (like the "exemplar" technique) helps the model understand the desired outcome more clearly.
Iterate and Refine Continuously:
Repeatedly test and adjust prompts.
Don’t accept the first result; keep tweaking for better quality.
Treat prompting as an ongoing conversation rather than a one-time command.
Avoid Ambiguity:
Bullet points and step-by-step instructions help avoid confusion.
Limit the number of tasks in a single prompt to keep focus.
Use the Model's Strengths:
Different models excel at different tasks. Use them accordingly:
Claude for creativity and analysis.
ChatGPT for broader search and task execution.
Perplexity for deep research and citations.
Leverage the AI for Prompt Writing:
Use the AI itself to improve and refine your prompts.
Ask the model for feedback to improve or simplify your prompt structure.
Prompt Length Management:
Be specific without overloading the model with unnecessary details.
Test how much detail is necessary for optimal results—sometimes less is more.
Prompt Analysis and Chain of Thought (CoT):
Encourage models to "think out loud" using internal monologues or analysis phases.
Separating tasks as in "analyze first, then respond" improves results, especially with complex reasoning.
Context Management:
If working on a long thread, summarize key points periodically to keep them in the model’s context window.
Ask the model to summarize the conversation to avoid losing track of important details.
Use Gravity Words Carefully:
Words like "story," "joke," or "metaphor" can heavily influence the output style.
Test how these words affect the results and adjust when necessary.
Prompting for Images and Video:
Use artistic language the model understands (e.g., lighting terms, cinematic framing).
Provide detailed descriptions for better visual results.
Utilize iterative refinement—start with a rough result and improve step by step.
Model Switching Strategy ("The Punt"):
If a model fails to deliver the desired result, switch to another (e.g., ChatGPT to Claude or Perplexity).
Cross-check results between models for accuracy and completeness.
Incorporate Search Features:
ChatGPT's improved search abilities reduce the need for providing multiple URLs.
Specify desired sources or types of information when using models with web search capabilities.
Subtasks and Multi-Step Prompts:
Break complex prompts into multiple subtasks for better performance.
Example: "First summarize, then compare, finally analyze."
Use Positive Framing:
Focus on telling the model what you want it to do rather than what to avoid.
Positive instructions yield better results than negative restrictions.
Prompt for Citations and Sources:
Explicitly request citations if you need sourced responses.
Be specific if the quality of references matters for the task.
Artifacts and Canvas Use:
Use tools like Canvas in ChatGPT and Artifacts in Claude for collaborative document editing.
These tools allow for clearer iteration and refinement directly within the workspace.
Automation and Chained Prompts:
Use tools like Respell, Make, or Zapier for chaining prompts together across different models.
Break down processes into multiple steps and automate them when possible.
Start Prompting—Don’t Overthink It:
The best way to get better at prompting is by doing it regularly.
Focus on experimenting and learning through hands-on practice.
Just Jokes

Did you know?
Microsoft plans to invest around $80 billion in AI-enabled data centers during fiscal year 2025, with more than half of this investment allocated within the United States. This significant expenditure underscores Microsoft's commitment to advancing AI and cloud-based applications, aiming to enhance infrastructure to support the growing demand for AI services.
In case you were wondering, $80 billion is roughly 33% of Microsoft’s reported revenue of $245 billion in 2024.
Clippy is living in a mansion being served by AI robots.
HEARD AROUND THE SLACK COOLER
What We Are Chatting About This Week Outside the Live Show
Florida judge put on a VR headset
Karl shared a story about a Florida judge who put on a VR headset in order to gain the perspective of a defendant who was charged with 9 counts of aggregated assault when he pulled a gun out and pointed it at people.
Karl thought it was ridiculous, but kind of neat while Beth commented “Today on AI WTF?” Brian (a Floridian) didn’t seem surprised at all this was happening in his own state and actually thought it was a new innovative way for reenactments to become part of court cases.
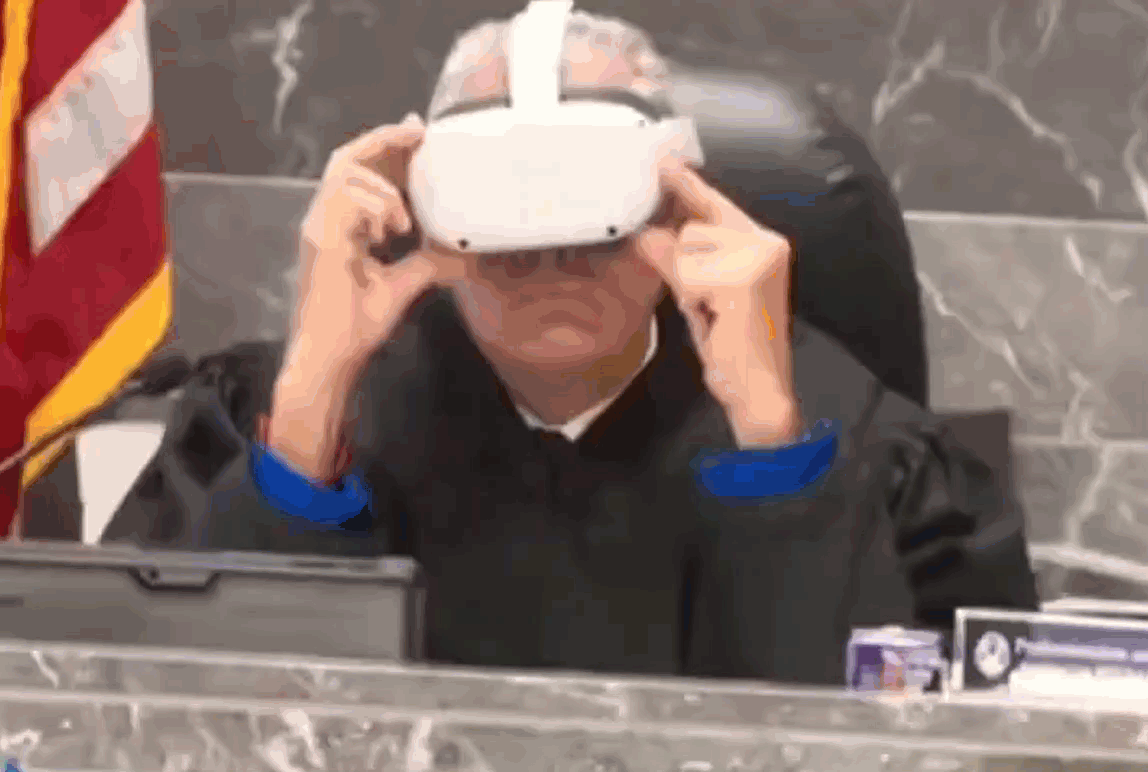
This Week’s Conundrum
A difficult problem or question that doesn't have a clear or easy solution.
The AI Memory Manipulation Paradox:
AI tools are emerging that can enhance memory recall or even generate synthetic memories—reconstructing past events or filling in gaps based on patterns from a person’s data. These technologies could help those with memory loss regain parts of their identity or allow people to experience vivid recreations of moments they barely remember.
However, this same capability raises profound ethical concerns: If AI can shape, alter, or even fabricate memories that feel authentic, does it compromise personal truth and reality?
The conundrum: If AI has the power to restore or enhance memories, but also to subtly alter or fabricate them, is it an act of healing or manipulation? Could such technology redefine personal truth for the better, or does it risk distorting a person’s sense of identity and lived experience?
News That Caught Our Eye
Osaka University Advances Android Facial Expression Technology
Osaka University has developed a new method for improving facial expressions in androids, using waveform models to create more natural emotional transitions. This innovation addresses the "uncanny valley" effect, where robots and avatars appear unsettlingly close to human but not quite right. By simulating gradual changes between emotional states, the androids can now shift expressions more naturally without requiring extensive programming.
Deeper Insight:
This research tackles one of the longest-standing challenges in human-robot interaction. Beyond robotics, this innovation could impact virtual reality avatars, gaming, and even AI-driven emotional support systems, where lifelike expressions are crucial for user comfort. If widely adopted, this could set a new standard for both physical and digital human interfaces.
NVIDIA Launches Jetson Thor for Humanoid Robots
NVIDIA unveiled the Jetson Thor, a powerful computing module designed specifically for humanoid robots. The device will power real-time AI functions, such as navigation and object manipulation, allowing robot manufacturers to build advanced machines without developing the core hardware themselves.
Deeper Insight:
Jetson Thor represents a significant shift from NVIDIA's typical GPU focus. By targeting robotics directly, NVIDIA is positioning itself as the backbone for a new generation of AI-powered physical systems. This could accelerate competition in humanoid robotics, especially for sectors like manufacturing, healthcare, and even home assistants.
NVIDIA Completes Acquisition of Run:AI
After nearly a year of regulatory delays, NVIDIA finalized its acquisition of Israeli AI infrastructure firm Run:AI. The deal strengthens NVIDIA’s position in AI workload management and resource optimization, focusing on data center efficiency and compute resource allocation.
Deeper Insight:
This acquisition is part of a broader trend where tech giants secure infrastructure control to dominate both hardware and software. NVIDIA's control over both the chips and now resource management could give it unmatched influence in enterprise AI deployments, though it raises antitrust concerns as the company continues to consolidate power.
ByteDance's $7 Billion NVIDIA Chip Investment Despite Sanctions
ByteDance plans to invest $7 billion in NVIDIA chips next year despite U.S. sanctions restricting chip sales to China. The company plans to bypass these restrictions by placing its data centers outside the U.S., avoiding direct export limitations.
Deeper Insight:
This move exposes the limitations of sanction strategies in slowing technological progress. China’s ability to work around restrictions highlights the globalization of AI infrastructure. This workaround could force policymakers to rethink export controls as companies find ways to source cutting-edge technology indirectly.
China’s DeepSeek V3 Model Impresses with Efficiency
DeepSeek V3, a 671-billion parameter mixture-of-experts model, has gained attention for activating only 5% of its parameters during inference, drastically cutting compute costs. The model competes with frontier models like GPT-4, delivering similar results at a fraction of the cost.
Deeper Insight:
This marks a shift from the "bigger is better" mindset dominating AI development. By focusing on selective activation, DeepSeek V3 proves that models can be both powerful and cost-effective. This could accelerate AI accessibility for smaller businesses and open-source developers, disrupting the dominance of high-cost, closed models like GPT-4.
Meta Announces AI-Powered Social Media Users
Meta plans to introduce millions of AI-generated users on Instagram and Facebook, complete with bios and interactive capabilities. These virtual personas are designed to boost platform engagement and interaction, mimicking real user behavior.
Deeper Insight:
This development blurs the line between organic and synthetic interaction. While it could provide personalized engagement, it also raises ethical concerns about user manipulation, data privacy, and the potential for algorithmic echo chambers. How Meta handles transparency around these AI profiles will be critical.
Hugging Face Launches Smolagents Library
Hugging Face released Smolagents, a lightweight open-source library designed to add agentic behaviors to language models. The tool simplifies the creation of AI agents capable of performing tasks autonomously with minimal code, focusing on efficiency and ease of deployment.
Deeper Insight:
Smolagents could democratize AI development by lowering the barrier for developers to create functional AI tools without large infrastructure costs. This positions Hugging Face as a leader in open-source AI accessibility and could inspire a wave of indie AI projects across various industries.
Meta Unveils Large Concept Model for Advanced Language Understanding
Meta released its Large Concept Model, a new approach to language modeling that predicts entire sentence structures rather than single tokens. This model, designed for multi-language support and concept-based reasoning, improves both generation speed and contextual accuracy.
Deeper Insight:
This leap in language modeling could redefine the foundation of how AI understands and generates text. By shifting from token prediction to sentence-level reasoning, models could become faster and more capable of complex language tasks like summarization, multi-turn conversations, and creative writing.
Cerebras and Sandia National Labs Train a Trillion-Parameter Model
Cerebras Systems and Sandia National Laboratories successfully trained a trillion-parameter model on a single CS3 wafer-scale engine. The technology consolidates memory and compute onto a single massive chip, vastly increasing speed and reducing latency for large-scale AI tasks.
Deeper Insight:
Cerebras’ breakthrough challenges the dominance of GPU clusters for large-scale AI training. If this architecture proves scalable, it could redefine how frontier models are developed, making massive AI models faster and cheaper to train without the need for vast server farms.
OpenAI Considers Becoming a Public Benefit Corporation
OpenAI announced plans to transition into a Delaware Public Benefit Corporation (PBC), a structure that allows balancing profit with public good. The move, however, has sparked controversy, with Elon Musk filing a lawsuit questioning the sincerity of the public benefit focus.
Deeper Insight:
This structural shift raises complex questions about whether for-profit incentives can genuinely align with public welfare in AI development. While the PBC model could offer flexibility, critics argue it might dilute OpenAI’s original mission of ensuring safe AI for all, especially given the increasing pressure for monetization in the AI space.
Did You Miss A Show Last Week?
Enjoy the replays on YouTube or take us with you in podcast form on Apple Podcasts or Spotify.